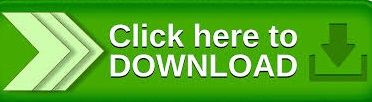
The patterns might not link back to any business outcome. Patterns are then detected in the dataset. This is a combination of business decisions, upfront engineering effort, and the application of some selection algorithms to create a clear, useful set of data that can be analyzed. The AIOps manifesto details five dimensions that align to form a valuable process of organizational learning. AIOps visualizes and surfaces this data, so it can be examined and, quite often, result in actionable insights. Traditionally, these things would be missed. Sudden changes in log volume, fluctuations in the number of background errors in an application, or a slowdown in latency that resolves itself. Rather than waiting until an issue has manifested itself in the form of an outage, you detect the subtle signs of a misbehaving system. The outcome of this constant analysis is simple. All of these “quirks” could be symptomatic of a larger issue that you simply haven’t found yet. It may be a sudden spike in logs from an application or an application that logs one error an hour suddenly fires 30 before settling back down again. Rather than relying on alerts we already know about, AIOps offers observability that can detect anomalies in your system that you haven’t found. (ART-STOCK-CREATIVE/Shutterstock) What is AIOps?ĪIOps leverages the immense power of artificial intelligence (AI) to detect issues. That means we typically find out about an issue at the same time as our users, or worse… after. If we haven’t thought of this error, we might have to wait until our users tell us. If we have already thought of this error, we might have alarms that tell us immediately. These errors tell us that something has broken. This may take the form of a sudden spike in HTTP 500 errors from our API, or it could be error logs from our database server. In the traditional method of operational improvement, we wait until our existing monitoring tells us that something has broken. It doesn’t attempt to get ahead of unknown issues, because that isn’t part of the cycle, and how would we implement fixes and improvements for an issue we don’t even know about yet? This model of operational improvement in the DevOps world is an “arms race.” We improve, a new type of bug comes along, and we improve again.
#CRITICAL OPS BACKGROUND HOW TO#
However, organizations like KPN, Google, COTY, and William Hill are learning how to break the cycle. This cycle is a powerful self-improvement model that allows organizations to keep up with their operational challenges as they scale and pursue their goals.
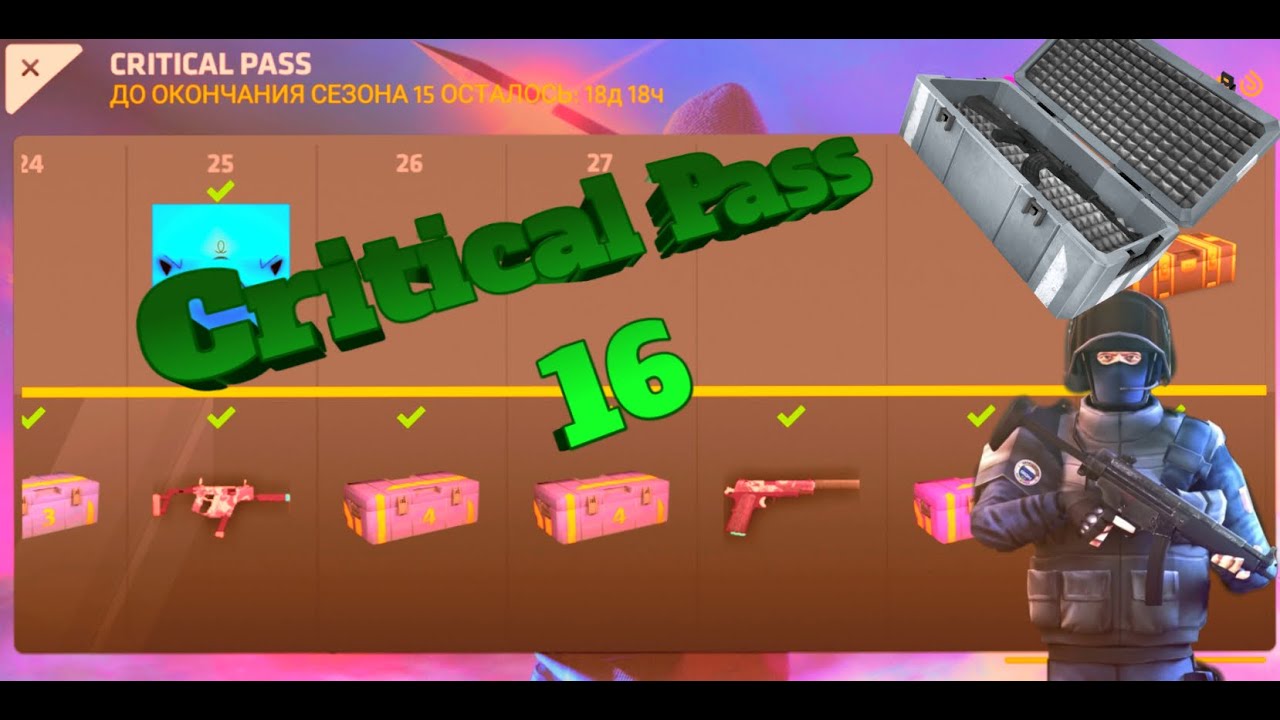
We detect an issue, remediate it, and put improvements in place to prevent it from recurring. When we consider operational challenges in the technologi field, it’s tempting to think of them as a continual battle.
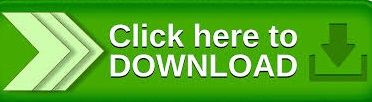